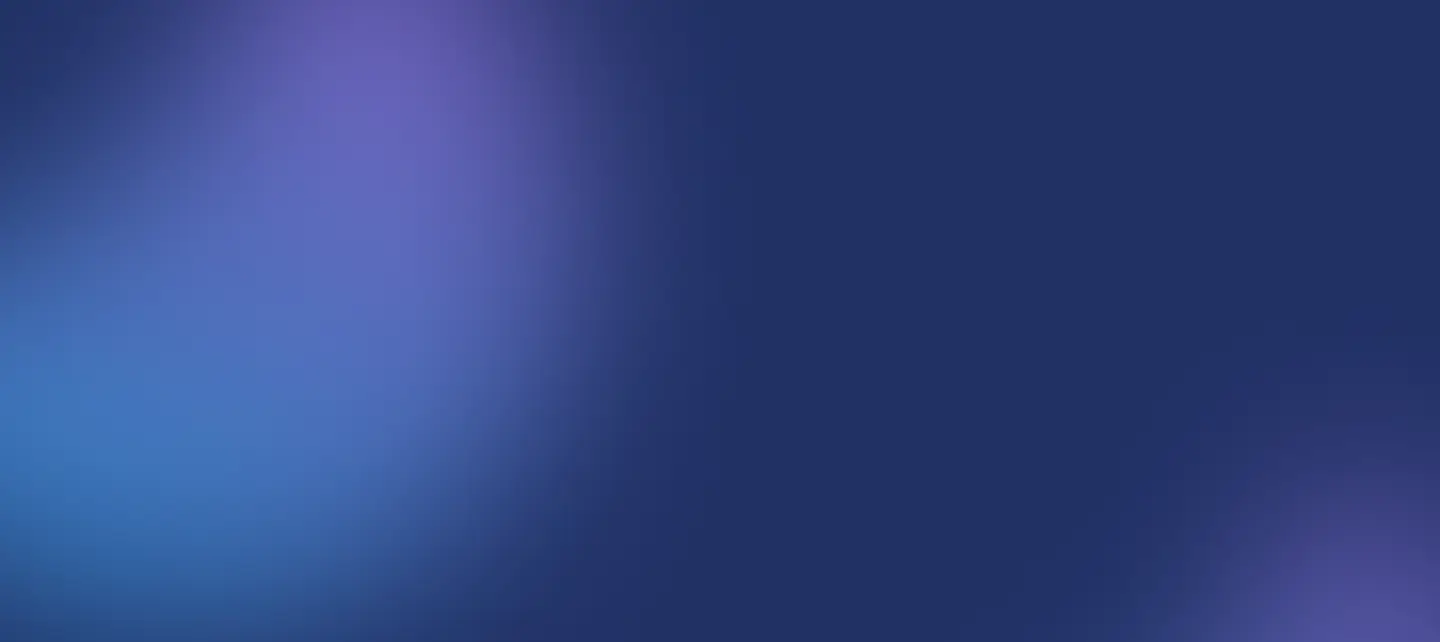
From Traditional Analytics to AI: 4 Ways Business Intelligence Has Evolved
5 April 2024
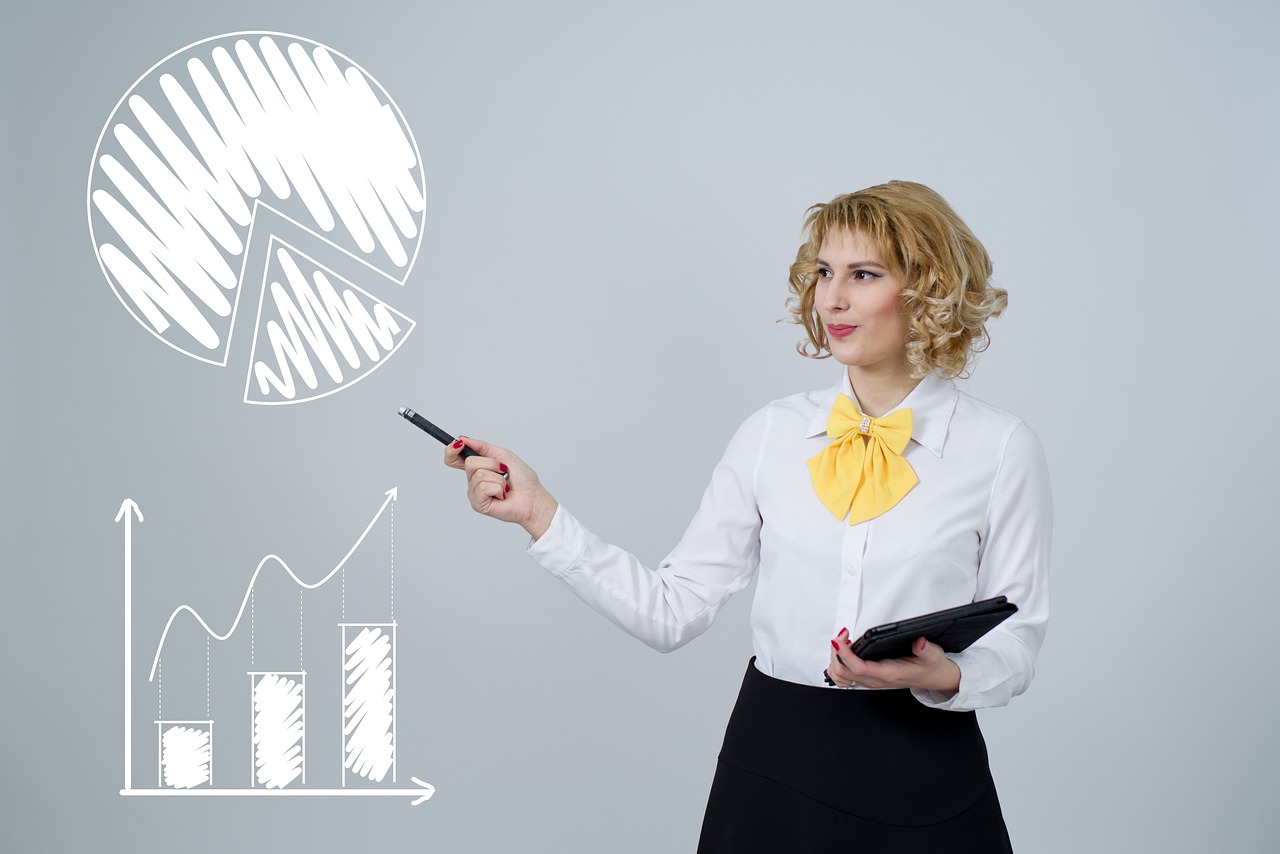
In the early days of business analytics, we were completely dependent on our tech departments to scope out statistical data, analyze the results, and report back to us.
This method, of course, had its limitations. Especially in terms of how much data our IT analysts could sift through, sort out, and summarize, at any one time. Yes, unlike today’s AI-assisted analytics, the manual data scraping, analyzing, and reporting of days gone by was time-consuming, menial, and most likely, exceptionally dull to complete. That is not to say that traditional analytics didn’t have its place, or provide value to businesses. Indeed, our historical approach to gathering, storing, and evaluating data is still considered the cornerstone of business intelligence, and it is the base from which our more modern methods have sprung.
Indeed, we’ve made quantum leaps in terms of what we can do with data with the advent of AI. But without traditional analytics, we wouldn’t have the foundation that has shaped the reality of what AI-assisted analytics looks like today. For this reason, although current students of coursework degrees in the field – such as a Master of Business Analytics online, for instance – are more likely to focus on the most up-to-date analytics innovations, understanding the evolution of business intelligence from its traditional roots can help us to contextualize our more recent knowledge.
Let’s discuss four ways our business intelligence has evolved into what it is today.
1. New Methods of Analysis: From Descriptive to Prescriptive
Looking back, we now have the hindsight to see that traditional business intelligence was preoccupied with uncovering and analyzing past outcomes – utilizing what was known as descriptive analytics. An example of descriptive analytics in action? Assessing how successful a completed marketing campaign was at reaching its target audiences, for instance, or how many sales of a particular product a recent advertisement drive generated.
But where traditional analytics excelled at painting a picture of results that had happened in the past, the integration of AI into BA has heralded the arrival of predictive, and even, prescriptive analytics. By observing patterns in statistical data, prescriptive analytics in particular can help businesses not only predict future outcomes, but also, offer recommendations on how to improve operations – either by mitigating potential risks, or even, maximizing opportunities.
The best part? This process can be further enhanced by another AI innovation: the recent development of machine learning.
2. The Development of Machine Learning: How Computers Make Decisions For Us
Thanks to the phenomenon that is machine learning – which has enabled computers to become more intelligent than ever before – AI-driven analytics can now help businesses not only review historical data but also hypothesize on how to do things better.
But how does AI do this? Machine learning enables AI software to develop an analytical ‘brain’ of sorts – facilitating its ability to identify patterns in data, and make informed recommendations based on these algorithms. As a result, machine learning can be instrumental in providing actionable advice for formulating more effective, data-informed business strategies.
In this sense, we can teach computers to make business decisions for us. Or at least, they can point us in the right direction regarding what future strategies we should be implementing.
If this is not the definition of modern-day business intelligence, we don’t know what is.
3. Data Storage and Sharing: How AI Makes it Better
Remember those archaic filing cabinets – beige, metallic, and full of crusty, decades-old documents – that used to line office walls in the ‘90s?
Fortunately, they are a relic of times past. Now, thanks to AI, we have far better – and more secure – ways to store our data. Namely, the Cloud, Google Drive, and myriad other digital file-sharing and storage programs.
4. AI Automation for Speedier Data Sourcing, Summarizing, & Analysis
Ah, AI automation. Quite honestly, if you can get a computer to do all of your mundane data collating and reporting for you, why wouldn’t you?
AI automation of data analytics allows for speedier, more efficient processes. The best part? You no longer need to get your tech team to manually sift through statistics.
If you’re wondering what that sound was – it was your analytics staff letting out an audible sigh of relief. By automating the more labor-intensive data tasks, you free up valuable time to get into the nitty-gritty: analyzing the insights and recommendations machine learning and AI-supported prescriptive analytics can provide.
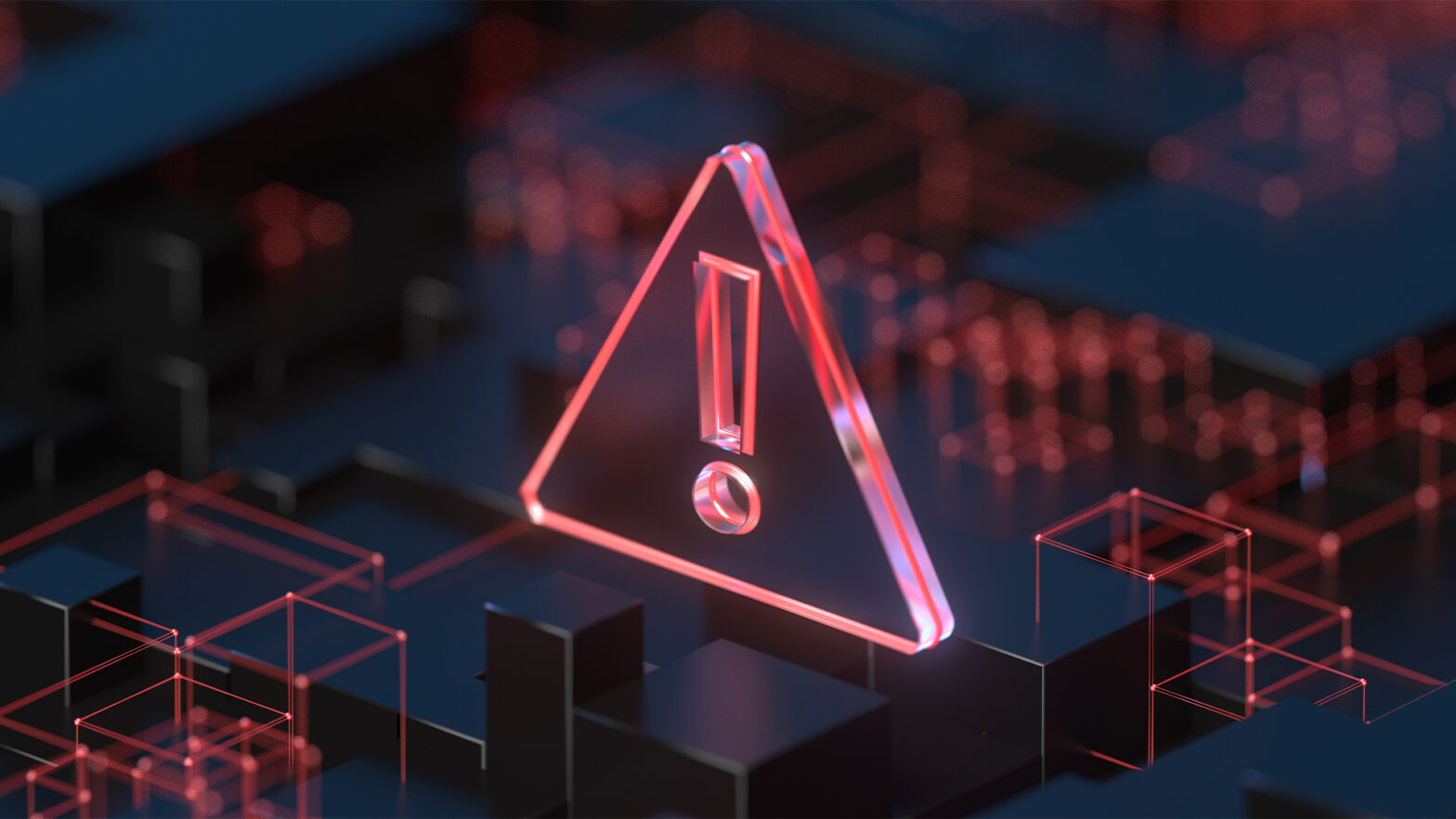
Technology
25 March 2025
Ransomware-As-A-Service Variants on the Rise With Critical Infrastructure Providers at the Greatest Risk
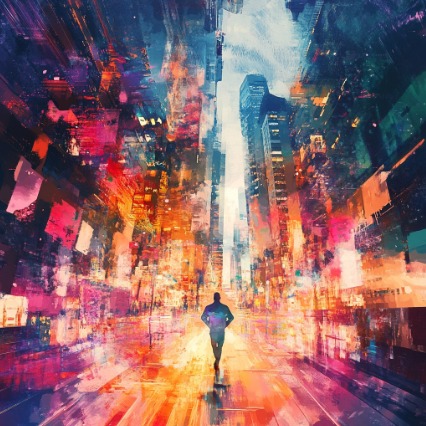
Business Advice
25 March 2025
Claims Processing Automation: How Insurers Can Cut Costs and Improve CX
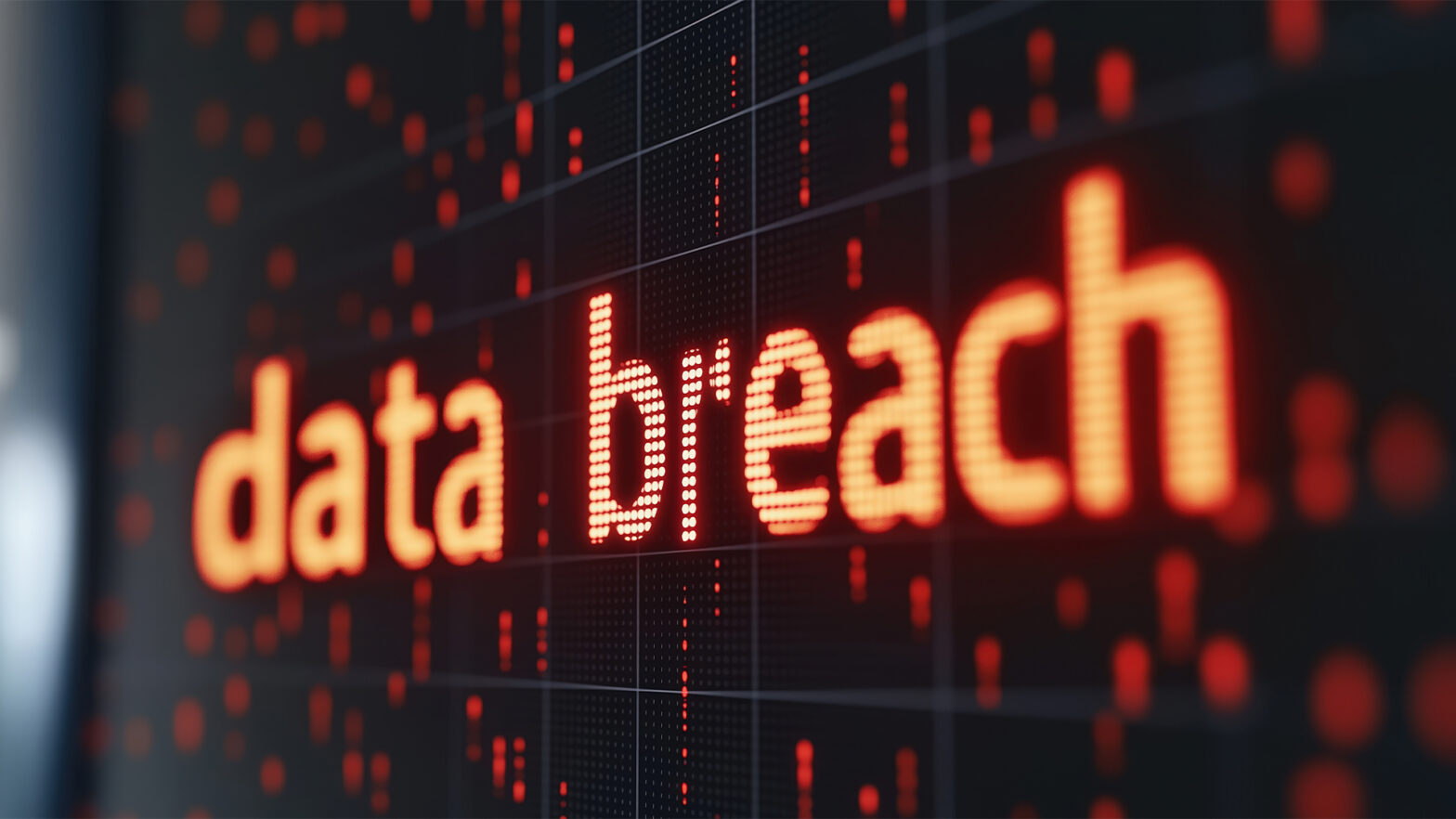
Technology
18 March 2025
Secret Signs Your Internet Security Has Been Compromised